

AUTOMA+ Pharmaceutical Automation & Digitalisation Congress 2025
Excited to sponsor this year's AUTOMA+ conference in our hometown Vienna, Austria. Our team will be on site and our CEO Harald Schnidar will discuss digital technologies for R&D.
EXPO 2025 in Osaka, Japan
Join Scarletred in the Austrian Pavillon again at the 2025 EXPO in Japan!
2025 AAD Annual Meeting
Meet Scarletred at our booth at the AAD annual meeting in Orlando, Florida.
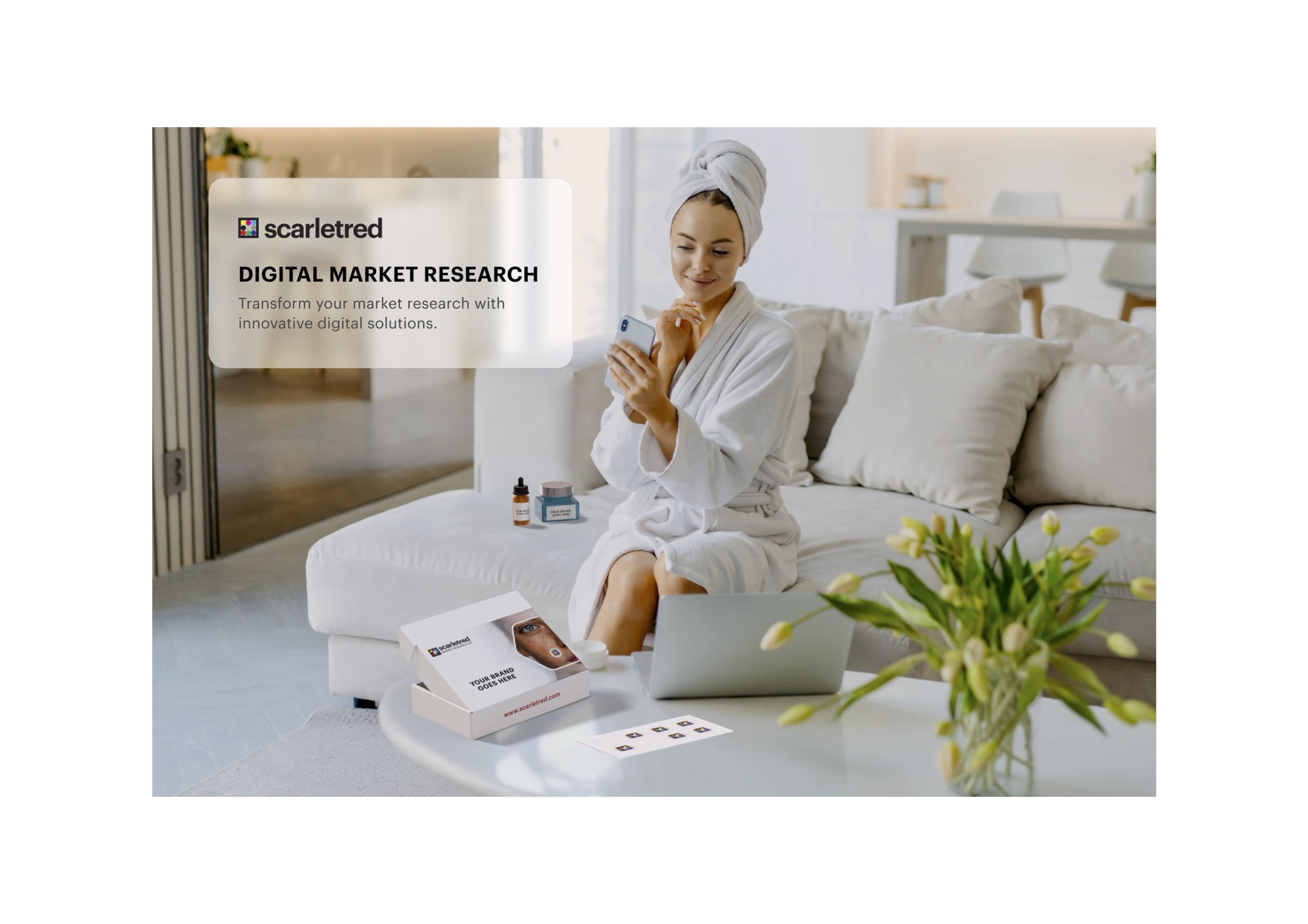
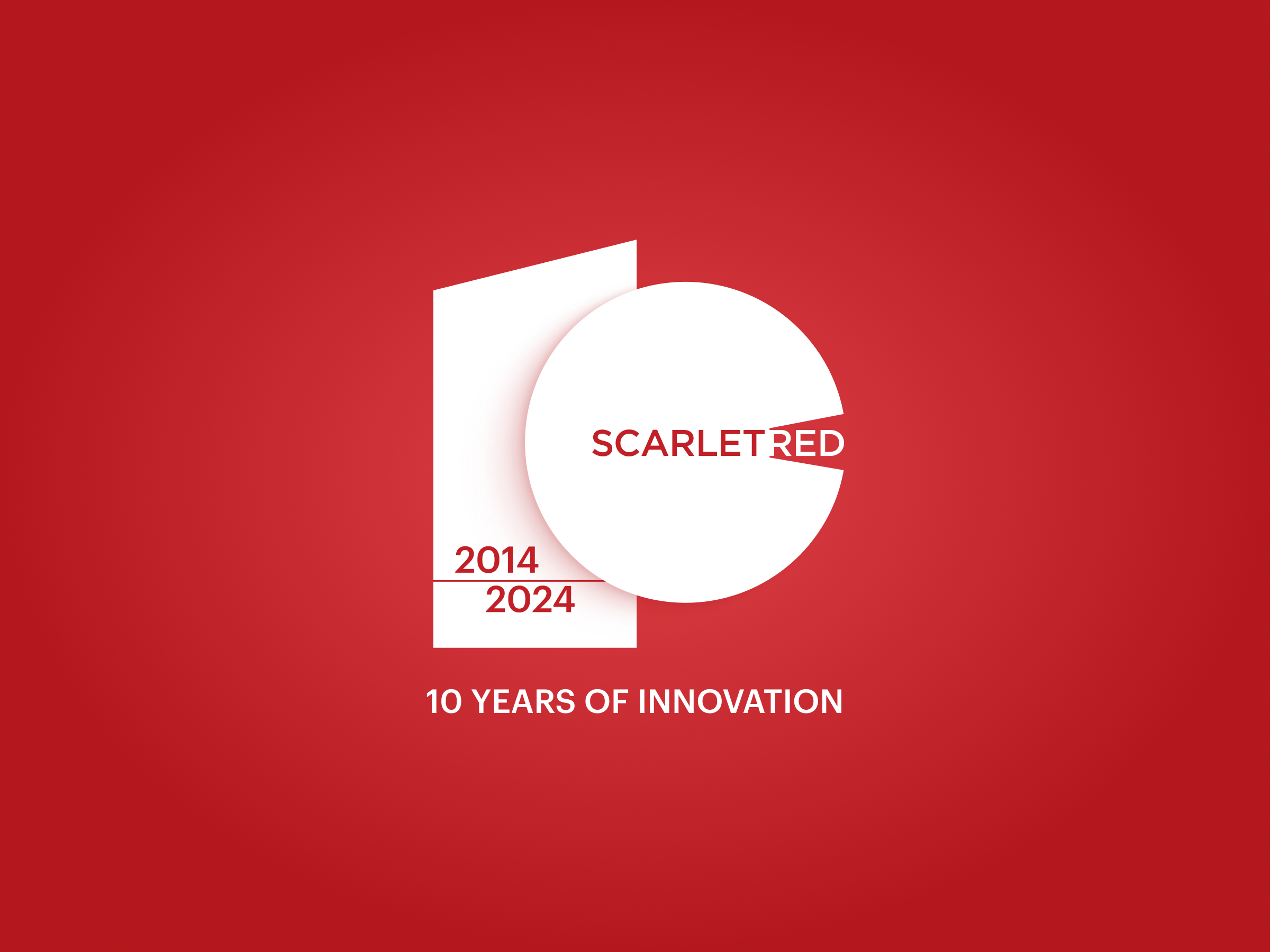
.jpeg)
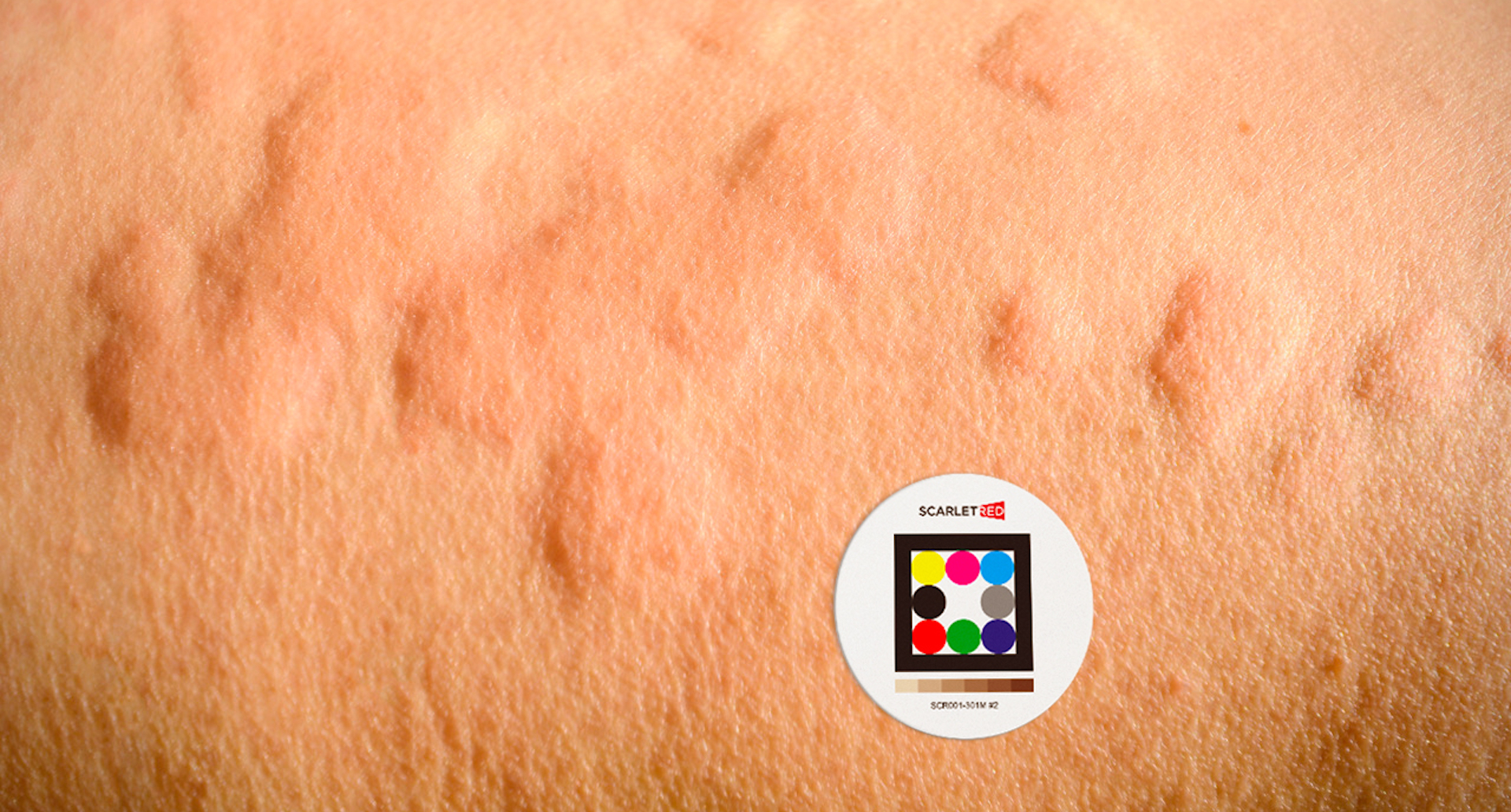
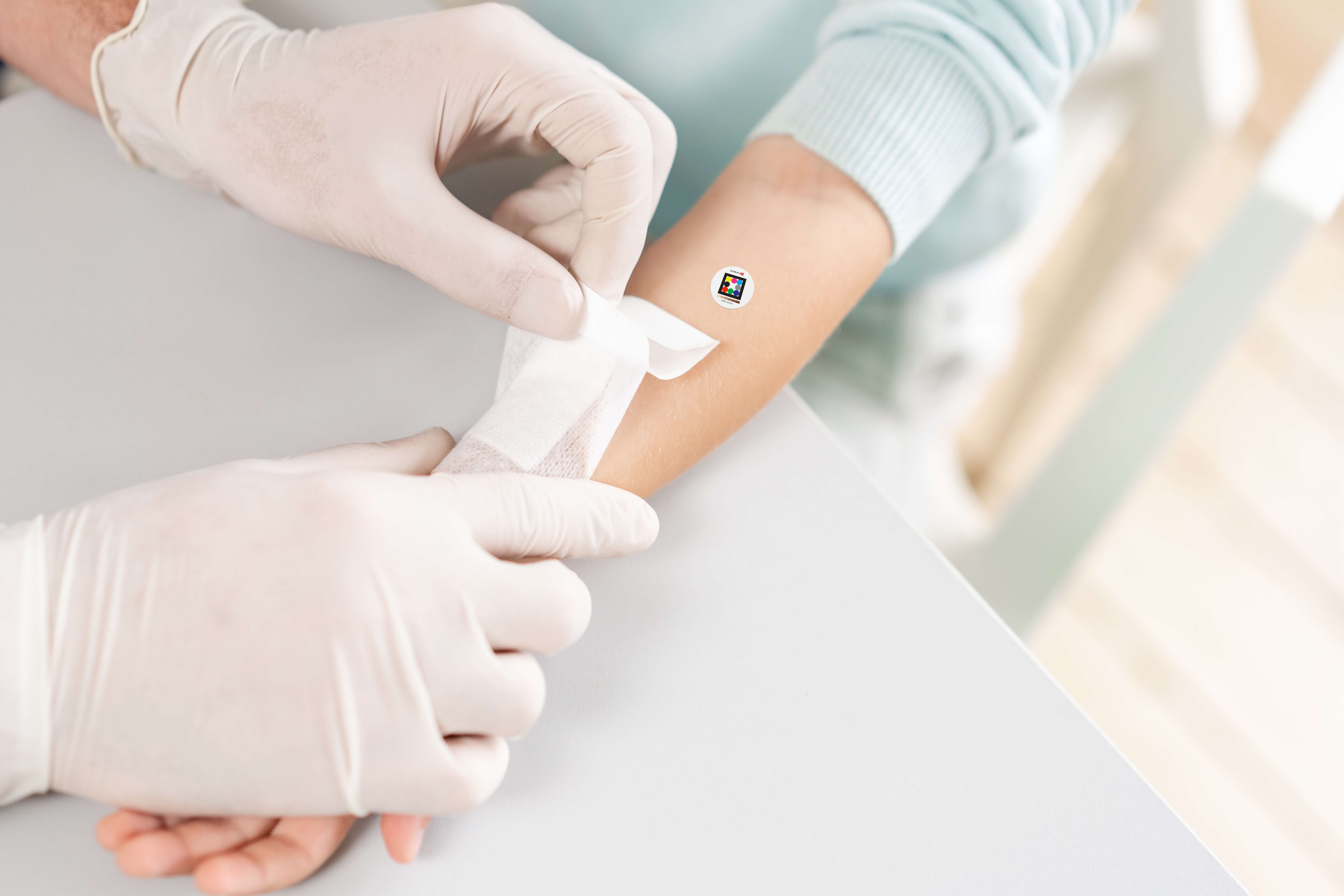
Decoding Hair Loss: AI-Enabled Analysis of Hair Growth and Follicle Dynamics as a Precision Medicine Tool For Alopecia
Background: Alopecia is a condition affecting millions of people worldwide, impacting not only physical health but also psychological well-being. Understanding the characteristics of hair and hair follicles is essential for developing effective treatments. In this study, we aim to analyze images of various areas to identify key parameters related to hair structure and distribution. Objectives: The main objective of this study is to develop an algorithm for analyzing hair images, exploring different shades and contrasts between hair and skin. This approach intends to provide a detailed quantitative assessment of the structural characteristics of hair and the distribution of hair follicles. Method: The images used in this study were acquired using the mobile application Scarletred®Vision, a validated and approved Software as a Medical Device (SaMD). The analysis includes the recognition of standardized SkinPatch sizes, used to deliver auto-color calibrated, high-resolution clinical images. A binary mask is then created to isolate the hair, allowing for contour detection and the removal of dimensional artifacts. Various parameters are calculated, including the length of the individual hair, as well as the average value, follicle positions, hair number per follicle, and hair thickness. Results: Preliminary results show that the patch sizes used for analysis were crucial for estimating the various hair parameters. Through this analysis, it was possible to estimate several significant parameters, including the average hair length, the total number of hairs present per analyzed area, and the frequency of multiple hairs within a single hair follicle, providing insights into follicular density. Additionally, hair thickness was evaluated in relation to patch sizes, contributing to a more comprehensive understanding of hair quality. A probabilistic estimate of the position of empty follicles was also conducted, suggesting areas potentially prone to alopecia. These results offer important insights into hair health and the mechanisms underlying hair loss. Conclusion: This study provides an innovative approach for analyzing hair and hair follicles through advanced image processing techniques. The results obtained can contribute to a better understanding of alopecia and the development of more targeted therapeutic interventions. Further research is needed to validate the models and deepen the analysis of the collected data.
Smart Imaging and Deep Learning for Objective Psoriasis Lesion Scoring: A Scarletred Proof-of-Concept Study
The assessment of psoriasis severity requires detailed, and robust lesion segmentation and tissue classification. This proof-of-concept study aimed to automate the classification of psoriasis disease severity on skin lesions using sophisticated deep learning algorithms. In this study, which was part of a randomized, controlled trial in psoriasis patients (NCT04394936), we used a total of 152 images of representative target plaques and 206 images of local body regions from 26 patients affected by psoriasis, both acquired with Scarletred®Vision, a clinically validated and approved Software as a Medical Device (SaMD) platform operating through an iOS-App on smartphones. As for the modeling aspect, we have trained a deep learning algorithm that employs target lesion scores derived from experts and manually delineated lesion areas. Our algorithm achieved high precision and accuracy in lesion segmentation, yielding a dice coefficient of 0.85. whereas for tissue classification, our model achieved a test accuracy and F-score of 97%. Additionally, our model estimated lesion severity for erythema, scaling and induration attaining correlations of 60%, 65% and 83% respectively between experts and AI scores, using a four-class prediction framework for grades 0 to 3. Similarly, our approach involved automatic body segmentation, enabling lesion localisation and estimation of affected body surface area. Notably, we demonstrated that successful AI classification was attained using a significantly reduced input dataset, showcasing a noteworthy advancement in rapid AI prototyping in clinical and hybrid trial environments, aimed at enhancing expert decision making.
AI powered Detection and Assessment of Onychomycosis: A Spotlight on Yellow and Deep Learning
Despite significant advances in computer-aided diagnostics, onychomycosis, a widespread fungal nail infection, lacks an automated approach for analysis and classification. The primary aim of our study was to develop and validate automated machine learning models for the accurate detection and classification of onychomycosis-aNected areas in toenails. The images used in this study were acquired using Scarletred®Vision mobile App under ambient light conditions, and all have uniform dimensions. Considering a total of 1687 unique images from 440 subjects, the research explores various degrees of onychomycosis and evaluates the extent of the infection in the detected toenails. We developed an advanced machine learning algorithm for precise segmentation and classification of onychomycosis-aNected toenails, utilizing expert annotations and advanced post-processing techniques. Additionally, an analysis of nail growth was performed, and a comparison graph with the percentage of infection was estimated. Using advanced machine learning algorithms, we successfully detected toenails, allowing for a detailed analysis of intricate structures within the images. We achieved a final validation loss of 0.0236 and an F1 score of 0.8566 for accurate toenail detection, while the Random Forest algorithm demonstrated 81% accuracy in classifying and distinguishing between infected and healthy areas of toenails aNected by onychomycosis. The superpixel method improved the algorithm's precision in identifying the infected regions, oNering promising advancements for decision support. Limited number of patients with darker skin color which may impact in the performance of the AI. While primarily examining the big toe’s toenail, our approach holds promise for extending and validating on a complete dataset, allowing broader assessments for onychomycosis. Our advanced machine learning algorithm shows enhancement in expert decision making.
Automated Classification of Hidradenitis Suppurativa Disease Severity by Convolutional Neural Network Analyses Using Calibrated Clinical Images
Abstract Background The assessment of hidradenitis suppurativa (HS) severity requires detailed, and error-prone lesion counts. This proof-of-concept study aimed to automatically classify HS disease severity using machine learning of clinical smartphone images. Methods 777 ambient-light and size-controlled images were used to build a class-balanced synthetic dataset (n = 7675). Convolutional neural networks (CNN) were used for automated severity classification (scale 0–3), and to assess disease-dynamics. International Hidradenitis Suppurativa Severity Score System (IHS4) served as reference. A U-NET algorithm was implemented for automated localization of diseased skin. Results CNNs were able to distinguish no/mild from moderate/severe disease with an overall prediction accuracy of 78% [receiver operating curve (AUC) 0.85]. Correct IHS4 classification was achieved with an overall accuracy of 72% (AUC 0.84–0.89). In addition, disease dynamics using IHS4 numerical values aligned with CNN outputs (NRMSE 0.262). The UNET algorithm localized lesions with a pixel accuracy of 88.1% and test loss of 0.42. Limitations Limitations in assessing tattooed and hairy skin. Limited number of patients with dark skin colour and Hurley I. Conclusion CNNs were able to distinguish no/mild from moderate/severe disease, classify disease severity over time, and automatically identify diseased skin areas and the skin phototype. This study breaks new grounds for fast, reliable, reproducible and easy-to-use HS severity assessments using clinical images.
Smart Imaging and Deep Learning for Objective Psoriasis Lesion Scoring: A Scarletred AI Proof-of-Concept Study
The assessment of psoriasis severity requires detailed, and robust lesion segmentation and tissue classification. This proof-of-concept study aimed to automate the classification of psoriasis disease severity on skin lesions using sophisticated deep learning algorithms. Despite remarkable progress in computer-aided diagnostics, there remains a scarcity of effective methodologies for analyzing and categorizing psoriasis. In this study, which was part of a randomized, controlled trial in psoriasis patients (NCT04394936), we proposed a robust pipeline to collect a dedicated, diverse and curated dataset. From 26 patients, we used a total of 152 images of representative psoriasis target plaques acquired with Scarletred® Vision, an innovative dermatologic software platform holds a CE class Im certification as a Medical Device and operates seamlessly through an iOS-App on smartphones. When paired with the Scarletred® Skin-Patch, it effectively standardizes the imaging procedure and facilitates the measurement of 2D/3D aspects, along with the evaluation of color and texture alterations in the skin. As for the modeling aspect, we have trained a deep learning algorithm that employs simultaneously collected target lesion scores derived from experts and manually delineated areas. This algorithm seamlessly integrates into the platform’s proprietary image augmentation pipeline. Our study findings reveal that the proposed model achieves high precision and accuracy across various tasks. This includes lesion segmentation, yielding a dice coefficient of 0.85 whereas for tissue classification, our model achieves a test accuracy and an F-score, of 88%, respectively. Furthermore, our model was able to estimate lesion severity for erythema, scaling and induration attaining test accuracies of 60%, 65% and 83% respectively by implementing a four-class prediction framework that covers grades 0, 1, 2, and 3. Notably, we demonstrated that successful AI classification has been attained using a significantly reduced input dataset compared to prior undertakings in this domain, showcasing a noteworthy advancement in efficiency and its use for prospective rapid AI prototyping in clinical and hybrid trial environments, with the objective of enhancing expert decision making.
From Pixels to Predictions: Development of a multimodal-based deep learning algorithm for accurate and efficient erythema score assessment in radiation induced dermatitis
Introduction & Objectives: Despite the significant progress made in computer-aided diagnostics using AI, there are currently limited viable methods available for the analysis and categorization of radiation-induced skin reactions (RISRs), making this study a critical step towards filling this gap. This study aims to develop advanced techniques that utilise a deep learning algorithm for the automatic classification of RISRs in accordance with the Common Terminology Criteria for Adverse Events (CTCAE) grading system. To achieve this objective, Scarletred® Vision, a state-of-the-art digital skin imaging method that allows for standardized monitoring and objective assessment of acute RISRs, was utilised. The study conducted SEV* measurements on 2D digital skin images after transforming them into the CIELAB colour space. Materials & Methods: In terms of data, we propose a robust pipeline to collect a dedicated, diverse, and curated image dataset using Scarletred® Vision, a CE certified medical device software platform. When it comes to models, we have trained a deep learning-based multimodal system that incorporates Scarletred® Vision augmentation pipeline. The dataset consisted of 2192 images, with a train-test size of 80:20 and image dimensions of 512 x 512. We used patch dimensions of 32 x 32 and 256 patches per image. The approach towards the solution involved input image patch, position embedding, and feature augmentation, with image augmentation based on feedback from ensembled prediction (R. Ranjan et al., 2021). Results: The results of our study indicate that the proposed model achieved high precision (92.51%), recall (91.21%), and F-score (91.83%), with a test accuracy of 92.02%. The model’s performance was evaluated in a class-wise manner, where the sensitivity for classes 0, 1, and 2 was found to be 96%, 94%, and 86%, respectively. Similarly, the specificity was determined to be 94%, 97%, and 97% for the respective classes. The overall accuracy for the model was found to be 96%, 94%, and 86% for classes 0, 1, and 2, respectively. These results indicate a high level of precision and discriminatory power of the model across all three classes. Conclusion: This study provides the first benchmark results using multimodal-based deep learning algorithm for erythema severity score classification. Data from this study highlights that the estimation of severity score for RISRs can be automated using Scarletred® Vision as decision support system. Our algorithm is currently being refined to provide also the estimated localisation, size and tissue composition of the lesion.
Wavelength-Dependent Effects of Photobiomodulation for Wound Care in Diabetic Wounds
Photobiomodulation, showing positive effects on wound healing processes, has been per- 13 formed mainly with lasers in the red/infrared spectrum. Light of shorter wavelengths can signifi- 14 cantly influence biological systems. This study aimed to evaluate and compare the therapeutic ef- 15 fects of pulsed LED light of different wavelengths on wound healing in a diabetic (db/db) mouse 16 excision wound model. LED therapy by REPULS was applied at either 470 nm (blue), 540 nm (green) 17 or 635 nm (red) at 40mW/cm2 each. Wound size and wound perfusion was assessed and correlated 18 to wound temperature and light absorption in the tissue. Red and trend-wise green light positively 19 stimulated wound healing while blue light was ineffective. Light absorption was wavelength-de- 20 pendent and was associated with significantly increased wound perfusion as measured by laser 21 Doppler imaging. Shorter wavelengths ranging from green to blue significantly increased wound 22 surface temperature, while red light, which penetrates deeper into tissue, led to a significant in- 23 crease body core temperature. In summary, wound treatment with pulsed red or green light re- 24 sulted in improved wound healing in diabetic mice. Since impeded wound healing in diabetic pa- 25 tients poses an ever-increasing socio-economic problem, LED therapy may be an effective,- easily 26 applied and cost-efficient supportive treatment for diabetic wound therapy.
Ivermectin Therapy for Rosacea: Software Platform to Quantify Changes in Erythema and Skin Texture
Introduction: Rosacea’s impact on quality of life comes from both visible and subjective symptoms. Ivermectin 1% cream has demonstrated efficacy and safety as a rosacea therapy, with both anti-inflammatory and acaricidal mechanisms of action. Objectives: Investigate the potential of ivermectin 1% cream to improve rosacea-associated erythema and invisible symptoms by combining established questionnaires with the photography and analysis platform Scarletred® Vision. Materials and Method: Single-center, open-label study of subjects (N=25) with moderate-severe rosacea (erythema, <10 papules/pustules, ≥15 Demodex mites/cm2) treated with ivermectin 1% cream once-daily for ≥16 weeks. Skin symptoms assessed at baseline, week 8 and ≥week 16; erythema assessed by clinician erythema assessment (CEA) and patient self-assessment (PSA); stinging, burning, dryness, itching severity assessed by questionnaire. Standardized digital skin images captured using a smartphone with Scarletred Vision App plus a Scarletred Skin Patch. Standard Erythema Value (SEV) algorithm used to quantify erythema, Gray Level Co-occurrence Matrix to quantify skin texture. Results: At baseline, mean CEA or PSA score was 3.6 with 44% score 3 and 56% score 4; subjects also had severe or moderate subjective symptoms. Ivermectin 1% cream significantly improved CEA and PSA, reduced lesions, and reduced Demodex counts (P<.0001 for all). Stinging, burning, dryness significantly reduced at 16 weeks (P<.0001) as was itching (P<.001). Scarletred Vision showed significant improvement of erythema (P<.0001) and skin roughness (P<.001) at ≥16 weeks, which agreed with CEA and PSA findings. Conclusions: Ivermectin 1% cream was efficacious in treating rosacea papules, pustules, and erythema; reducing Demodex counts, and improving subjective skin symptoms.
Topical Bimiralisib Shows Meaningful Cutaneous Drug Levels in Healthy Volunteers and Mycosis Fungoides Patients but No Clinical Activity in a First-in-Human, Randomized Controlled Trial
Mycosis fungoides (MF) is a subtype of CTCL with a low incidence and high medical need for novel treatments. The objective of this randomized, placebo-controlled, double-blinded, first-in-human study was to evaluate safety, efficacy, cutaneous and systemic pharmacokinetics (PK) of topical bimiralisib in healthy volunteers (HVs) and MF patients. In this trial, a total of 6 HVs and 19 early-stage MF patients were treated with 2.0% bimiralisib gel and/or placebo. Drug efficacy was assessed by the Composite Assessment of Index Lesion Severity (CAILS) score, supported by objective measuring methods to quantify lesion severity. PK blood samples were collected frequently and cutaneous PK was investigated in skin punch biopsies on the last day of treatment. Local distribution of bimiralisib in HVs showed a mean exposure of 2.54 μg/g in the epidermis. A systemic concentration was observed after application of a target dose of 2 mg/cm2 on 400 cm2, with a mean Cavg of 0.96 ng/mL. Systemic exposure of bimiralisib was reached in all treated MF patients, and normalized plasma concentrations showed a 144% increased exposure compared to HVs, with an observed mean Cavg of 4.49 ng/mL and a mean cutaneous concentration of 5.3 μg/g. No difference in CAILS or objective lesion severity quantification upon 42 days of once-daily treatment was observed in the MF patient group. In general, the treatment was well tolerated in terms of local reactions as well as systemic adverse events. In conclusion, we showed that topical bimiralisib treatment leads to (i) meaningful cutaneous drug levels and (ii) well-tolerated systemic drug exposure in MF patients and (iii) a lack of clinical efficacy, in need of further exploration due to numerous unknown factors, before depreciation of topical bimiralisib as a novel therapeutic drug for CTCLs.
Ivermectin Treatment in Rosacea: How Novel Smartphone Technology Can Support Monitoring Rosacea-Associated Signs and Symptoms
Background: Rosacea lessens patients’ quality of life not only by visible symptoms like erythema, papules, and pustules but also by invisible symptoms like stinging, burning and dryness. Ivermectin 1% cream has recently been introduced as an efficient therapy for papules and pustules in rosacea patients. Objectives: To investigate the potential of ivermectin 1% cream to improve rosacea- associated erythema and invisible symptoms by combining established questionnaires with the novel photography and analysis tool Scarletred® Vision. Methods: We performed an open monocentric pilot study including 25 Caucasian patients presenting with moderate to severe rosacea with erythema, less than 10 papules and/or pustules, and ≥15 Demodex mites/cm2. Patients applied 1 g of ivermectin 1% cream (Soolantra®) once a day for ≥ 16 weeks. Skin symptoms were recorded at baseline, week 8 and ≥ week 16. Grade of erythema was determined by clinician erythema assessment (CEA) and patient self-assessment (PSA). Severity of invisible skin symptoms (stinging and/or burning, dryness, itching) were assessed by questionnaire. Erythema and skin texture were additionally quantified using Scarletred® Vision. Results: Ivermectin 1% cream significantly reduced invisible symptoms of rosacea (stinging and/or burning, dryness: p<0.0001; itching p<0.001; at ≥ 16 weeks). Analysis with Scarletred® Vision confirmed CEA and PSA results for improvement of erythema (p<0.0001; at ≥ 16 weeks) and skin roughness (p<0.001; at ≥ 16 weeks). Conclusions: Treatment with ivermectin 1% cream is efficient in treating not only rosacea- associated papules and pustules but also erythema and invisible skin symptoms.
Automated Classification of Hidradenitis Suppurativa Disease Severity by Convolutional Neural Network Analyses Using Clinical Images
Assessment of Hidradenitis suppurativa (HS) severity requires details, time-consuming and error-prone lesion counts. To address the need for objective assessments this study aimed to ease severity assessment by automated machine learning based classification using clinical cell-phone images.
Sensitivity and Specificity of SARS-CoV-2 Rapid Antigen Detection Tests Using Oral, Anterior Nasal, and Nasopharyngeal Swabs: a Diagnostic Accuracy Study
The objective of our study was to evaluate the sensitivity and specificity of rapid antigen detection tests versus those of reverse transcriptase PCR (RT-PCR) using oral, anterior nasal, and nasopharyngeal swabs. The underlying prospective, diagnostic case-control-type accuracy study included 87 hospitalized and nonhospi- talized participants in a positive and a negative sample cohort between 16 March and 14 May 2021 in two hospitals in Vienna. SARS-CoV-2 infection status was con- firmed by RT-PCR. Participants self-performed one oral and one anterior nasal swab for the rapid antigen test, immediately followed by two nasopharyngeal swabs for the rapid antigen test and RT-PCR by the investigator. Test results were read after 15 min, and participants completed a questionnaire in the meantime. Test parameters were calculated based on the evaluation of 87 participants. The overall sensitivity of rapid antigen detection tests versus that of RT-PCR with oral, anterior nasal, and nasopharyn- geal samples was 18.18% (95% confidence interval [CI] 8.19% to 32.71%), 63.04% (95% CI 47.55% to 76.79%), and 73.33% (95% CI 58.06% to 85.4%), respectively. All sampling methods had a test specificity of 100% regardless of the cycle threshold (CT) value. Rapid antigen detection tests using self-collected anterior nasal swabs proved to be as sensitive as and more tolerable than professionally collected nasopharyngeal swabs for CT values up to 30 determined by RT-PCR. This finding illustrates the reliability of tests obtained by adequate self-collected anterior nasal specimen. Sensitivity was depend- ent upon the CT value for each sampling method. While the main advantage of rapid antigen detection tests is the immediate availability of results, PCR should be preferred in crucial settings wherever possible.
The mathematics of erythema: Development of machine learning models for artificial intelligence assisted measurement and severity scoring of radiation induced dermatitis
Although significant advancements in computer-aided diagnostics using artificial intelligence (AI) have been made, to date, no viable method for radiation-induced skin reaction (RISR) analysis and classification is available. The objective of this single-center study was to develop machine learning and deep learning approaches using deep convolutional neural networks (CNNs) for automatic classification of RISRs according to the Common Terminology Criteria for Adverse Events (CTCAE) grading system. Scarletred(R)Vision, a novel and state-of-the-art digital skin imaging method capable of remote monitoring and objective assessment of acute RISRs was used to convert 2D digital skin images using the CIELAB color space and conduct SEV* measurements. A set of different machine learning and deep convolutional neural network-based algorithms has been explored for the automatic classification of RISRs. A total of 2263 distinct images from 209 patients were analyzed for training and testing the machine learning and CNN algorithms. For a 2-class problem of healthy skin (grade 0) versus erythema (grade ≥ 1), all machine learning models produced an accuracy of above 70%, and the sensitivity and specificity of erythema recognition were 67–72% and 72–83%, respectively. The CNN produced a test accuracy of 74%, sensitivity of 66%, and specificity of 83% for predicting healthy and erythema cases. For the severity grade prediction of a 3-class problem (grade 0 versus 1 versus 2), the overall test accuracy was 60–67%, and the sensitivities were 56–82%, 35–59%, and 65–72%, respectively. For estimating the severity grade of each class, the CNN obtained an accuracy of 73%, 66%, and 82%, respectively. Ensemble learning combines several individual predictions to obtain a better generalization performance. Furthermore, we exploited ensemble learning by deploying a CNN model as a meta-learner. The ensemble CNN based on bagging and majority voting shows an accuracy, sensitivity and specificity of 87%, 90%, and 82% for a 2-class problem, respectively. For a 3-class problem, the ensemble CNN shows an overall accuracy of 66%, while for each grade (0, 1, and 2) accuracies were 76%, 69%, and 87%, sensitivities were 70%, 57%, and 71%, and specificities were 78%, 75%, and 95%, respectively. This study is the first to focus on erythema in radiation-dermatitis and produces benchmark results using machine learning models. The outcome of this study validates that the proposed system can act as a pre-screening and decision support tool for oncologists or patients to provide fast, reliable, and efficient assessment of erythema grading.
A Global Industry and Market Research Report on Injection Site Reactions
Injection site reactions (ISRs) are a constellation of symptoms, such as erythema, swelling, pain, and induration, occurring at the site of the injection. Documenting and monitoring ISRs are integral components of the clinical trial process for any injectable drug or treatment. During clinical development, local reactions at the injection site must be tracked to complete the safety assessment of the investigational product. ISRs can occur right after the substance has been administered and subsequently evolve over time after the individual has left the health care provider, highlighting the need for a solution that can accommodate real-time, remote, home-based monitoring. Medical professionals agree that traditional methods of ISR monitoring in the product development phases are lacking, leading to a loss of time, precision, and data. The global digitalization trend and the impact of the current SARS-CoV-2 pandemic are the main driving forces behind the development of new injectables, revealing the urgent requirement for novel state-of-the-art tools that can be more efficiently implemented. In the present global market and research study, we (SCARLETRED) give an overview of the applicable industries and our technological solution, Scarletred®Vision, which outperforms conventional methods and is on its way to becoming the modern standard for remote ISR Monitoring and objective quantification in a broad range of application fields.
Digital Assessment of Hidradenitis Suppurativa Disease Activity
Hidradenitis suppurativa (HS) is a chronic inflammatory skin disease affecting up to 2% of European adults. Disease activity is commonly assessed by counting of inflammatory nodules, abscesses and fistulas.
Testing the feasibility of augmented digital skin imaging to objectively compare the efficacy of topical treatments for radiodermatitis
Radiation-induced dermatitis (RID) is routinely graded by visual inspection. Inter-observer variability makes this approach inadequate for an objective assessment of the efficacy of different topical treatments. In this study we report on the first clinical application of a new image-analysis tool developed to measure the relevant effects quantitatively and to compare the effects of two different topical preparations used to treat RID.
Local Therapy Response Already Predictable by Day 3
The present case report shows that an innovative technology -Scarletred® Vision - can predict the response of the topical application of Enstilar® foam already by day 3.
128 SHADES OF RED: Objective Remote Assessment of Radiation Dermatitis by Augmented Digital Skin Imaging
The purpose of our investigation was to develop a novel and state of the art digital skin imaging method capable for remote monitoring and objective assessment of Radiation Induced Dermatitis (RID). Therefore, radiation therapy related side effects were assessed by medical experts according to Common Terminology Criteria for Adverse Events (CTCAE) grade of severity in 20 female breast cancer patients in a clinical trial over the treatment time frame of 25-28 radiation cycles, 50.0 – 50.4 Gy each. Furthermore the intensity of developed skin erythema was documented by using conventional spectrophotometry plus digital skin imaging. Thereby we could derive the Standardized Erythema Value (SEV), a novel objective parameter, which in contrast to single parametric L* and a* delivers a long dynamic measurement range for analyzing RID from bright to very dark skin tones. Methodical superiority of the SEV could be proven over spectrophotometer measurements in terms of a higher sensitivity and by enabling signal intensity mapping in analyzed skin images. Our thereupon-derived patent enables novel objective dermatologic eHealth applications in a broad range of medical and industrial use by opening likewise the window for augmented dermatology. The first of its kind system is now already further developed in form of the medical device product Scarletred®Vision. It is available on the market for primary usage in clinical trials and in medical routine.